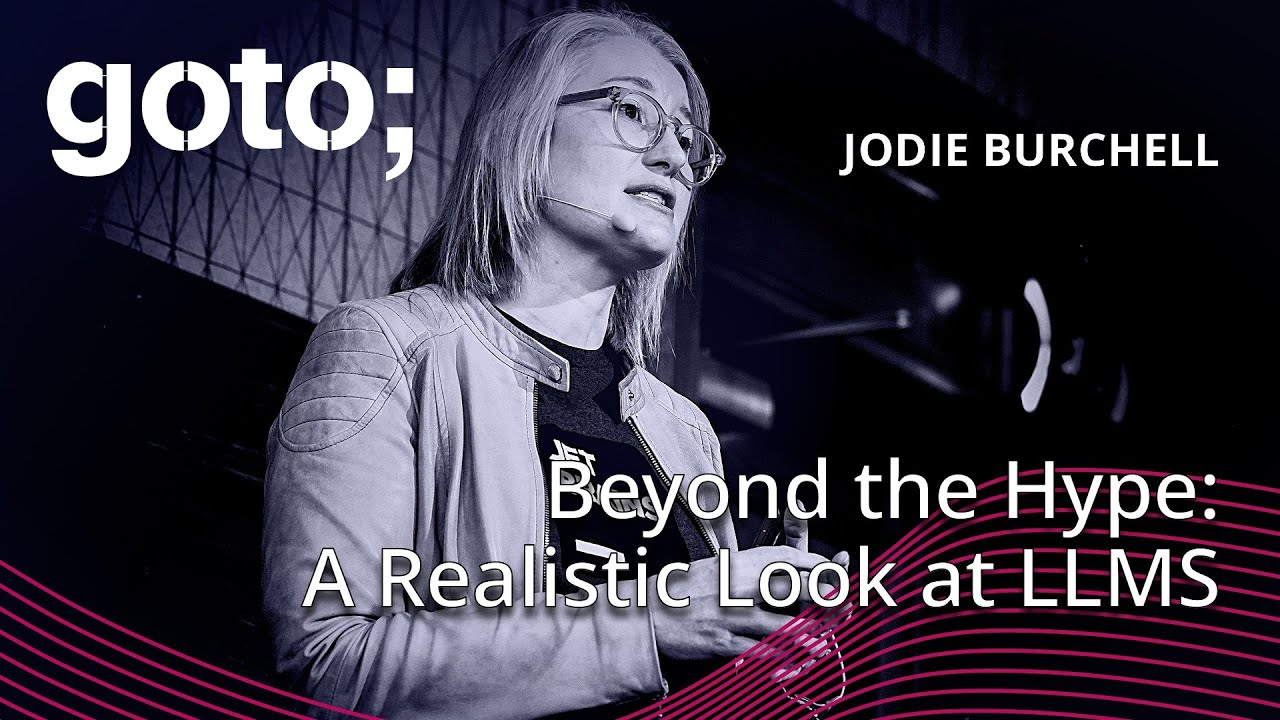
Beyond the Hype: A Realistic Look at Large Language Models
You need to be signed in to add a collection
If you've been remotely tuned in to the latest developments in large language models (LLMs), you've likely been inundated with news, ranging from claims that these models will replace numerous white-collar jobs to declarations of sentience and an impending AI apocalypse. At this stage, the hype surrounding these models has far surpassed the actual useful information available. In this talk, we’ll cut through the noise and delve deep into the current applications, risks, and limitations of LLMs. We’ll start with early research endeavours aimed at creating an "artificial brain" and trace the path that has led us to today's sophisticated text models. Along the way, we'll address how these models have been mistaken for intelligent systems. We’ll shed light on the actual requirements for developing true artificial general intelligence, and see how far LLMs are from this goal. We’ll end with a practical demonstration of how you can use LLMs in a way that plays to their strengths, by showing you how to build a system which leans into these models’ powerful natural language capabilities.
Transcript
If you've been remotely tuned in to the latest developments in large language models (LLMs), you've likely been inundated with news, ranging from claims that these models will replace numerous white-collar jobs to declarations of sentience and an impending AI apocalypse. At this stage, the hype surrounding these models has far surpassed the actual useful information available.
In this talk, we’ll cut through the noise and delve deep into the current applications, risks, and limitations of LLMs. We’ll start with early research endeavours aimed at creating an "artificial brain" and trace the path that has led us to today's sophisticated text models. Along the way, we'll address how these models have been mistaken for intelligent systems.
We’ll shed light on the actual requirements for developing true artificial general intelligence, and see how far LLMs are from this goal. We’ll end with a practical demonstration of how you can use LLMs in a way that plays to their strengths, by showing you how to build a system which leans into these models’ powerful natural language capabilities.