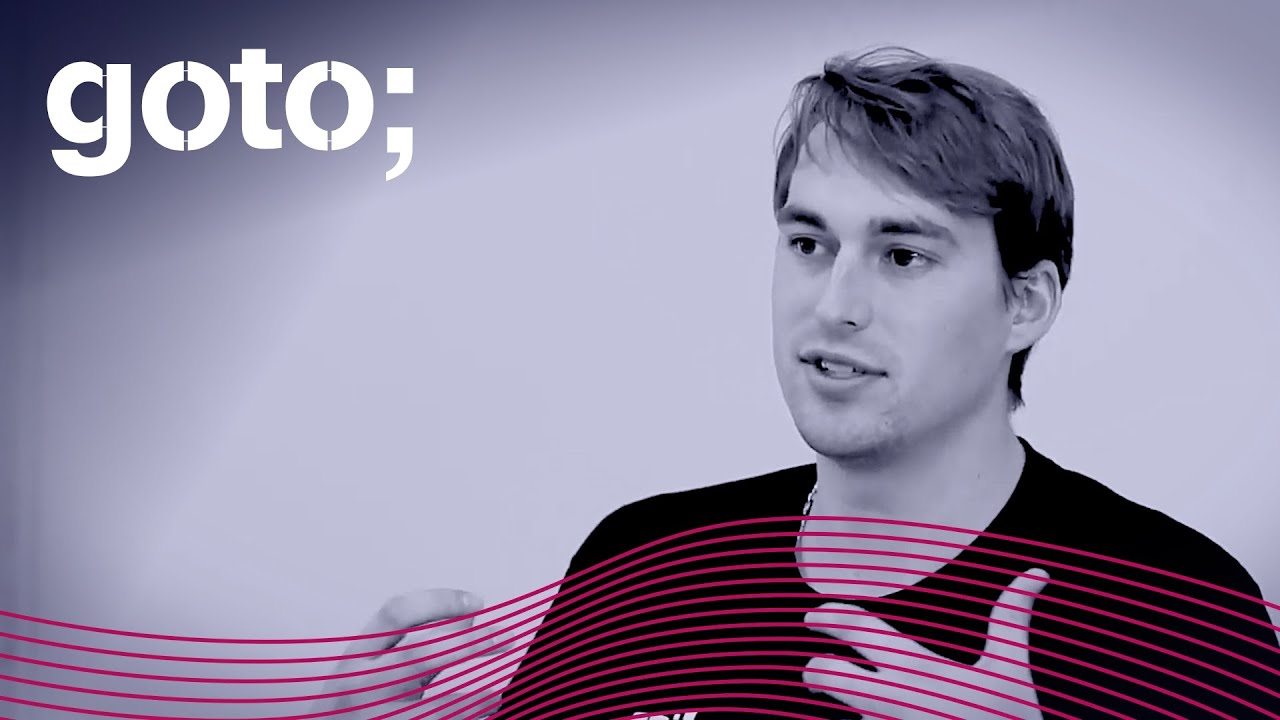
Small is the New Big: Designing Compact Deep Learning Models
You need to be signed in to add a collection
The emergence of deep neural networks (DNNs) in recent years has enabled ground-breaking abilities and applications for modern intelligent systems. State-of-the-art DNNs have been found to achieve high accuracy on tasks in computer vision and natural language processing, even outperforming humans on object recognition tasks. Concurrently, the increasing complexity and sophistication of DNNs is predicated on significant power consumption, model size and computing resources. For example, since 2012, the training complexity of AI models has increased by 350,000x. These factors have been found to limit deep learning’s performance in real-time applications, in large-scale systems, and on low-power devices. Furthermore, many low-end and cost-effective devices do not have the resources to execute DNN inference, causing users to sacrifice privacy and offload processing to the cloud. Application developers, software engineers and algorithm architects must now create intelligent solution that deal with strict latency constraints, such as in smart city, mobility and healthcare applications which often require that inference be performed in a matter of milliseconds, often with limited hardware. To do so, we will take a look at promising new ways of using AI to help human experts design highly compact, high-performance Deep Neural Networks on cloud and edge devices.
Transcript
The emergence of deep neural networks (DNNs) in recent years has enabled ground-breaking abilities and applications for modern intelligent systems. State-of-the-art DNNs have been found to achieve high accuracy on tasks in computer vision and natural language processing, even outperforming humans on object recognition tasks. Concurrently, the increasing complexity and sophistication of DNNs is predicated on significant power consumption, model size and computing resources. For example, since 2012, the training complexity of AI models has increased by 350,000x. These factors have been found to limit deep learning’s performance in real-time applications, in large-scale systems, and on low-power devices.
Furthermore, many low-end and cost-effective devices do not have the resources to execute DNN inference, causing users to sacrifice privacy and offload processing to the cloud. Application developers, software engineers and algorithm architects must now create intelligent solution that deal with strict latency constraints, such as in smart city, mobility and healthcare applications which often require that inference be performed in a matter of milliseconds, often with limited hardware.
To do so, we will take a look at promising new ways of using AI to help human experts design highly compact, high-performance Deep Neural Networks on cloud and edge devices.