Jesse Anderson on The Who, What, When, Where, and How of Data Project Success
Data projects have gained significant attention in recent years, with businesses eager to harness the power of data analytics and AI. However, despite the enthusiasm, a staggering 85% of big data projects fail to make it into production, according to Gartner. This dismal statistic raises several questions: Why do data projects fail at such an alarming rate? What are the common pitfalls that organizations encounter in their data-driven endeavors? How can software engineers and data professionals improve the odds of success? In this talk during the YOW! London 2022 conference, Jesse Anderson delved into the technical aspects of why most data projects fail and revealed the key questions that must be addressed to avoid failure.
The Technology Trap
One common misconception in the world of data projects is that technology is the silver bullet that can solve all problems. Software engineers often find themselves embroiled in debates over which tools and platforms to use. Should it be Snowflake or Databricks? Python or Java or Rust? The truth is, technology is undoubtedly important, but it is just one piece of the puzzle. Relying solely on technology without addressing more fundamental aspects can lead to project failure.
Moreover, selecting the right technology stack is a critical decision in any data project. The choice should not be solely driven by whitepapers from cloud vendors or aggressive marketing campaigns. Cloud vendors are naturally biased towards their own offerings, and their recommendations may not align with the specific needs of your project.
Data teams should have clear architectural plans that dictate where and how data will be processed. The decision-making process should involve experienced data engineers who can provide unbiased advice on technology choices, ensuring they align with the project's goals and requirements.
Asking the Right Questions
To steer data projects toward success, it's essential to ask the right questions. Who, what, when, where, and how are fundamental queries that need answers. By focusing on these questions, many technology-related issues can be resolved. Rather than beginning with a specific technology in mind, start with a deep understanding of the project's requirements and objectives. Then, choose the appropriate technology stack that aligns with these needs.
a. Who - Success begins with having the right people on your team. This includes data scientists, data engineers, and operations personnel. Overloading data scientists with data engineering tasks can hamper productivity.
b. What - Merely stating that a project aims to leverage AI or data analytics is far from sufficient. A clear, actionable plan for value creation must be in place. It should specify how data will be used to achieve tangible outcomes for the business.
c. When - Having a well-defined timeline for generating value is vital. Projects that promise results in unrealistically short or excessively long timeframes often face failure. Showing incremental value along the way can help maintain stakeholder support.
d. Where - While technology is a component, it's essential to have a clear architectural strategy. Avoid blindly following vendor recommendations or adopting technologies without understanding their suitability for your project's goals.
e. How - Overloading a project with too many tasks simultaneously can lead to chaos. Instead, focus on executing a few critical tasks effectively. This promotes success and avoids getting bogged down in multiple directions.
Most importantly, it’s important to ask "why is your data valuable?" This question will always be posed by stakeholders evaluating project costs. It's crucial to establish an ROI for your data expenditure. A good rule of thumb is aiming for a 10x ROI and, ensuring that the benefits significantly outweigh the costs. Communicate in terms of business outcomes and money saved or earned rather than technical details. This not only justifies the project's existence but also safeguards it from being deemed superfluous during economic downturns.
Balancing Data Teams
A crucial factor that often leads to project failure is an imbalance within data teams. For example, having an abundance of data scientists but a shortage of data engineers spells trouble. Data scientists possess strong analytical skills but may lack the engineering expertise required to implement solutions at scale. Conversely, data engineers are proficient in handling data infrastructure but may not possess the mathematical and statistical knowledge needed for advanced data analysis.
The recommended ratio for a well-rounded data team is approximately five to ten data engineers per data scientist. This inversion of the commonly seen ratio highlights the intensive nature of data engineering, especially in machine learning and AI projects. Achieving the right balance among data scientists, data engineers, and operations personnel is critical for project success.
Avoiding the ‘Field of Dreams’ Fallacy
Data projects often fall victim to unrealistic expectations, including the "Field of Dreams" fallacy, which assumes that "if you build it, they will come." Having a clear timeline for when value will be generated is crucial. "When it's ready" is not a viable timeframe, and overly ambitious deadlines can set projects up for failure.
A realistic timeline should be established, one that considers the project's complexity and the capabilities of the team. Infeasible timeframes mandated by higher-ups can lead to disappointment and frustration. Clear communication with stakeholders about what is achievable within a given timeframe is essential.
Essentially, data project failures are far too common in the software engineering and data science domains. While technology plays a role in these setbacks, the root causes often lie in organizational, strategic, and human factors. Addressing the right questions, balancing data teams, defining business value, making informed technology choices, and setting realistic timelines are key steps toward project success.
In an era where data-driven insights are vital for business growth and innovation, it is imperative for organizations to take a holistic approach to their data projects. By doing so, they can significantly improve the chances of joining the elusive 15% of projects that succeed in delivering tangible value and innovation.
CONTENT
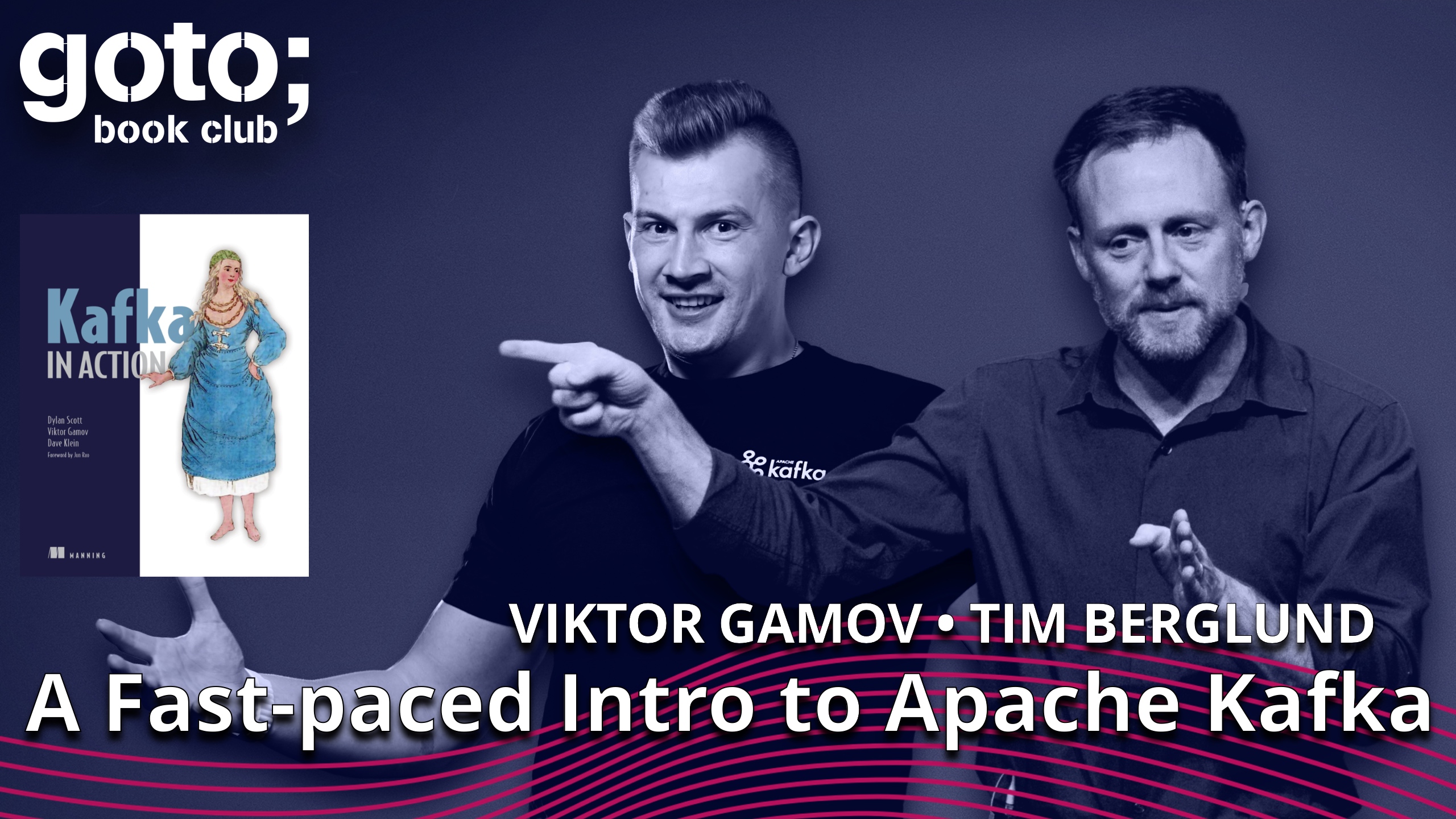
Kafka in Action
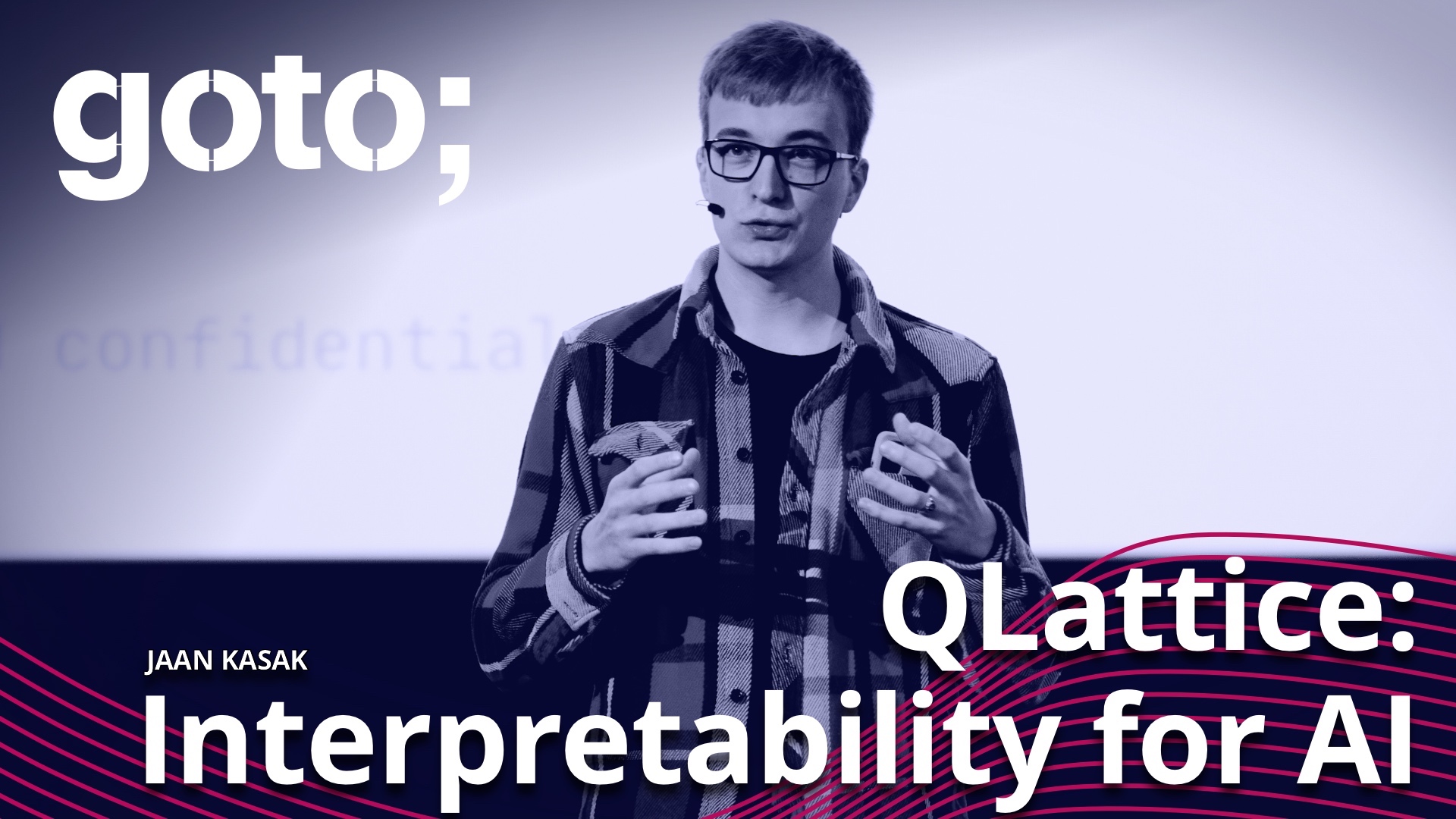
Abzu’s Pioneering Technology, the QLattice®, Introduces a New Standard of Interpretability to Artificial Intelligence
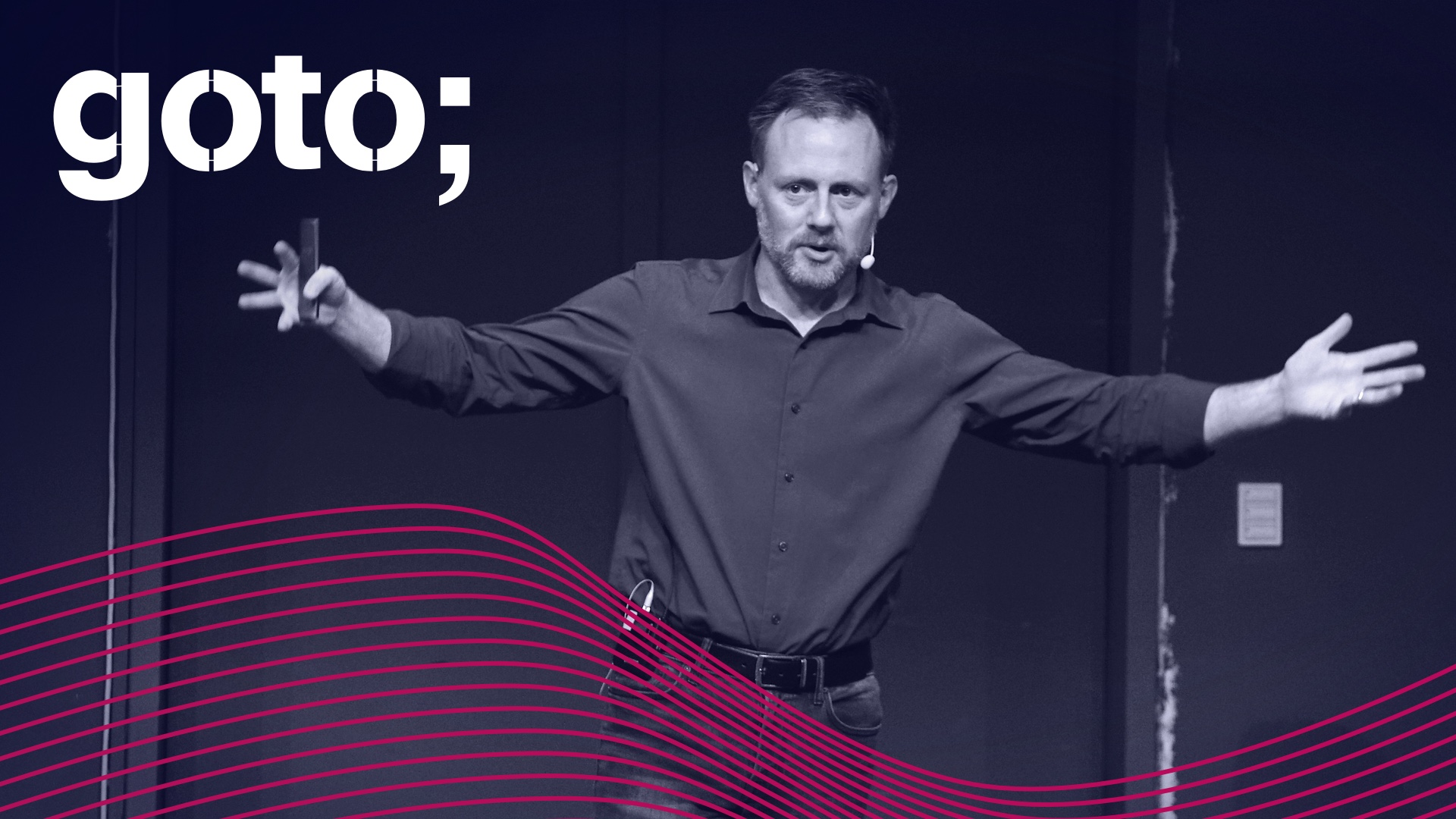
Processing Streaming Data with KSQL
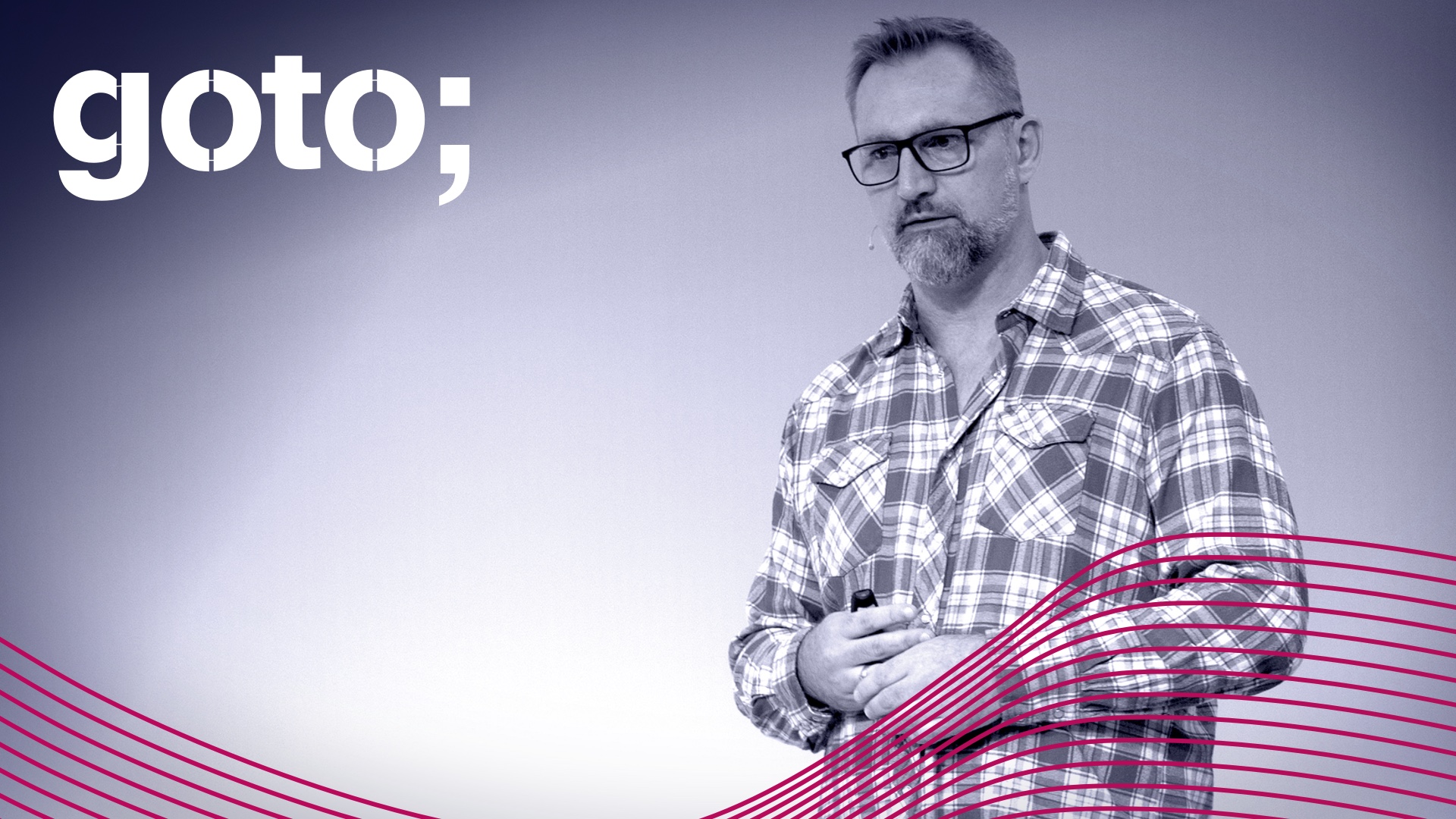